IT Blog | A Successful Warehouse Digitization Requires Reliable Data | Part 2/2
Deriving Recommended Courses of Action from Valid Material Master Data
‘Data is the new oil’. This now widely used quote was coined by Meglena Kunevas back in 2009. With regard to oil, certain developments such as combustion engines or specialty chemicals were required in order to make full use of this precious resource. Similar considerations apply to the warehouse, where data runs all ongoing processes. But it takes more than just data. Only if the quote is extended with ‘...but information is the new gold’, can the circle be successfully closed. Ultimately, the goal is to derive actions from data on the basis of information.
Especially in warehouse logistics, this virtualized alchemy – the enrichment of data into information – can be excellently observed. A storage location search, for instance, is only reliable if the algorithm has sufficiently accurate knowledge of the respective stock level and the current situation in the warehouse. Of course, information such as the filling rate, the number of cases to be stored, or the number of cases requested from an aisle or shuttle level are also of great importance to an analysis of the warehouse performance values. This information is even more essential when, for every step of the case storage process, a result for the way forward should be obtained in real time. This ideally allows the entire storage system to continue to run steadily instead of provoking an overload in a certain area.
Obviously, selecting a case to fulfill an order in an automated warehouse can only work if the following information is consistent, up-to-date and reliable: current storage location, content, and quantity, as well as possible restrictions (reservations or blocks by another case). This is taken for granted and considered a given basic functionality of a WMS system without ever being mentioned in the scope of supply.
Precise Warehousing Results in Reliable Data
Perfectly tuned warehouse management relies on an extensive, complete and current set of data. For a WMS to achieve its full functionality, it must rely on valid processes, a precise warehouse management by the warehouse staff, and the use of relevant data.
Performance Loss Due to Lack of Data Analysis
Missing data or a lack of data analysis inevitably leads to performance losses in the warehouse. For example, orders from the warehouse cannot be processed at a speed comparable to that of the competition. Additionally, the storage range of individual products drops to a few hours, since the inconsistent and outdated parameterized values for the stock management no longer correspond to the current requirements. And yet, the warehouse is full when visually inspected because enormous quantities of the wrong products occupy rack space, which is urgently needed.
Adjusting Stock Management to the Order Structures
These are the unfortunate effects of a stock management that is not aligned with actual order structures. This can have several causes:
The wrong model has been selected for optimal stock management.
Once selected, the model has never been extended or adjusted.
The underlying data of the stock management has never been updated.
Especially in times of e-commerce and online shops as well as the global interconnectedness of customers via social media, the classic stock management models, which are documented in literature, become more and more obsolete. These classic models are based on coincidental purchases. However, can you still call it a coincidence today if several customers (unexpectedly) order the same product simultaneously? Or is it now more and more predictable if certain social media platforms, including their influencers, are closely followed as data sources?
Data Maintenance and Metadata Management – An Investment in the Future
In order to obtain an answer to the question, one has to focus on the order data. Only this can provide information on the following decisions:
The right logistics concept.
The appropriate and correct stock management model.
The optimal parameterization (at the time of observation).
The indicators from the market, which in case of an excess/shortfall of stock requirements, trigger a reconsideration of the model and/or parameters.
Let's take a look at a ‘what-if analysis’ of the recent past regarding the following question: What if I had already readjusted my model/parameters several months ago or made the recommended change in item assignment? Due to the observed order data, it is possible to obtain a rather significant impression of the untapped efficiency and performance potential. In view of the increasing market dynamics, the time periods for reconsiderations are becoming shorter and shorter. To start only after the structural problem has been identified is far too late. Especially when it comes to site (re)plannings, missing data strikingly contradicts the optimal planning result with regard to the concept and the sustainability of the new warehouse site.
Increasing Efficiency and Reducing Logistics Costs
A future without any steps towards digitization is hard to imagine. Apart from the strategies that were already outlined in previous blog articles, state-of-the-art algorithms allow for even more complex approaches. The possibilities to increase efficiency, which were mentioned in these blog articles, significantly reduce the share of logistics costs.
There are already a number of exemplary companies who have reacted early to make active use of their data and the generation of facts by means of automated, data-run decision mechanisms. A decision that has proven successful. This enables them to not only react to changes in a less cost-intensive and faster way, but also lets them benefit from the market dynamics which are made transparent by such data.
This procedure is recommended by SSI SCHAEFER in any case, since an active use of customer data through the automated decision mechanisms mentioned above can take the performance of the customer warehouses to a whole new level. If you are interested, do not hesitate to contact SSI SCHAEFER as your partner.
About the author:
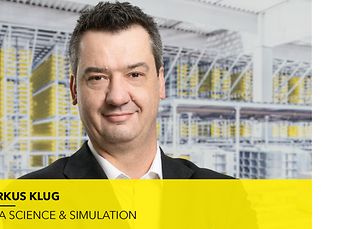
Markus Klug graduated from the TU Wien in Applied Mathematics. He did some postgraduate research in Glasgow regarding Kernel-based Methods and their area of possible applications for event-discrete simulation models. Afterwards he managed national and international research and innovation projects related to transport logistics, site logistics and worldwide supply chains at the applied industrial research center Seibersdorf.
Markus Klug has been part of SSI SCHAEFER since 2013 and is responsible for the use of data analysis and simulation, a role which later grew to encompass data science and artificial intelligence/machine learning.