Predictive Maintenance: Avoiding Downtimes Thanks to Smart Maintenance
Storing, conveying, picking: In complex logistics processes, all components have to interact perfectly. What happens if one of the components fails? Hurry! Re-establishing a smooth flow of goods requires an unplanned service intervention. This incurs costs – and they rise with every minute of downtime.
There is an option to avoid this: predictive maintenance.
What is predictive maintenance?
Predictive maintenance is a method to predict the maintenance requirements of a machine. For this purpose, operating data is gathered in real-time and analyzed using additional information and intelligent algorithms. Anomaly detection helps to detect wear and tear and impending failures at an early stage and plan maintenance work in advance before problems arise.
Maintenance strategies compared
Predictive maintenance is a proactive approach that aims to carry out maintenance and repairs as early as possible – but only when they are actually necessary. In this respect, predictive maintenance differs from other maintenance types:
Reactive maintenance
Maintenance and repair work are only carried out when faults occur or when
the wear and tear is well advanced.
Preventive maintenance
Inspections and maintenance work are carried out at regular intervals, regardless
of the actual condition of the system.
Condition-based maintenance
Condition-based maintenance is also reactive, but based on machine data.
Maintenance is carried out as soon as threshold values have been reached.
The 5 steps of predictive maintenance
Predictive maintenance facilitates data-based predictions about the condition of a system and enables you to plan maintenance measures accordingly. Where do you get this information from? How do you determine the optimum time for maintenance? What are the technical requirements?
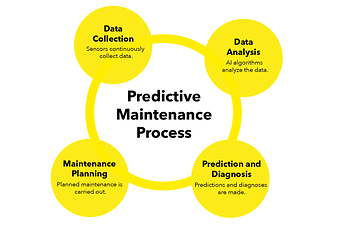
Step 1: Data collection
In the first step, operating data is recorded using sensors on the machines and
stored in a database via an IoT connection. For this purpose, SSI SCHAEFER uses
the “Nano Box”, a standard IPC by Siemens to gather real-time data at control
level and transfer it to the Computerized Maintenance Management System,
the WAMAS Maintenance Center.
Step 2: Data analysis
The gathered data is then combined with other relevant information. Catchword: Big Data. In addition to the individual maintenance history available in the WAMAS Maintenance Center, SSI SCHAEFER also uses global machine data. On this basis, special data analysis algorithms and machine learning technologies can recognize patterns and anomalies.
Step 3: Predictive diagnosis
Statistical methods and models are used to create predictive diagnoses of the future condition of machines and components based on the analysis results. Artificial intelligence is also used. The system constantly learns from new data and delivers increasingly precise predictions.
Step 4: Maintenance planning
The created forecasts provide information about the ideal time and type of maintenance work required. This allows maintenance work to be planned in advance so that spare parts can be ordered in good time and downtimes can be avoided.
Step 5: Maintenance procedure
Maintenance work can be carried out particularly efficiently on the basis of previous planning – for example, during off-peak times and with material and personnel expenditure tailored to requirements.
Why does predictive maintenance pay off?
Predictive maintenance provides companies with planning security, reduces maintenance effort and ensures smooth material flows. The data-based and AI-supported maintenance strategy can increase the productivity and efficiency of an entire system – and save significant costs.
Reducing unplanned maintenance operations is one of the biggest advantages of our predictive maintenance solution. This helps avoid costly downtimes and allows resources to be used particularly efficiently.
Predictive maintenance does not only increase availability, but also helps to extend the useful life of the machines and systems. Data analysis helps identify problems at an early stage before major damage occurs. Wear and tear can also be minimized through demand-oriented maintenance. Long-term investments are thus protected and the sustainability of the plant is improved.
Does predictive maintenance also have disadvantages?
The successful implementation of predictive maintenance also entails some challenges. After creating the necessary infrastructure for the communication of AI systems, the selection and application of suitable analysis platforms and AI models require specialized know-how. The data quality is also crucial – a lack of information can lead to inaccurate predictions and incorrect maintenance decisions.
The best solution for predictive maintenance
Could predictive maintenance be too complex, especially for small and medium-sized companies? Not if you have a suitable partner by your side. SSI SCHAEFER provides tailor-made predictive maintenance solutions from a single source. With state-of-the-art technologies and personal support from experts, you can benefit from all the advantages of predictive maintenance.
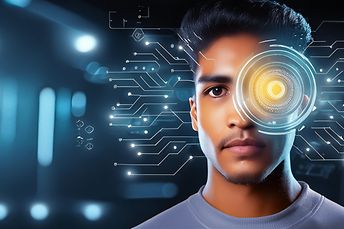
About the author
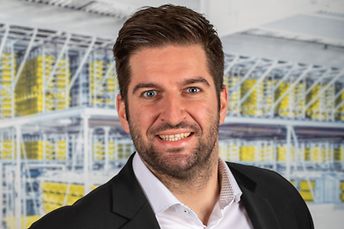
Stefan Unterberger can draw on over ten years of experience in project management and more than four years of strategic expertise: At SSI SCHAEFER, he previously was in charge of IT projects, the Global Project Management Office as well as Strategy & Business Transformation. Stefan Unterberger has focused on this expertise since January 2024 in his position as Manager for Products & Innovation, where he is driving the development of the unique predictive maintenance solution.